A Probabilistic Approach for Integrating Heterogeneous Knowledge Sources
Dutta, Arnab
;
Meilicke, Christian
;
Ponzetto, Simone Paolo
![[img]](https://madoc.bib.uni-mannheim.de/style/images/fileicons/application_pdf.png) 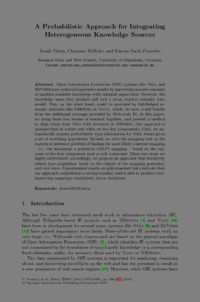 Vorschau |
|
PDF
chp%3A10.1007%2F978-3-319-07443-6_20.pdf
- Veröffentlichte Version
Download (293kB)
|
URL:
|
https://madoc.bib.uni-mannheim.de/36461
|
Weitere URL:
|
https://madata.bib.uni-mannheim.de/65/
|
URN:
|
urn:nbn:de:bsz:180-madoc-364610
|
Dokumenttyp:
|
Konferenzveröffentlichung
|
Erscheinungsjahr:
|
2014
|
Buchtitel:
|
The Semantic Web: Semantics and Big Data : 11th International Conference, ESWC 2014, Anissaras, Crete, Greece, May 25-29, 2014, Proceedings
|
Titel einer Zeitschrift oder einer Reihe:
|
Lecture Notes in Computer Science
|
Band/Volume:
|
8465
|
Seitenbereich:
|
286-301
|
Veranstaltungsdatum:
|
25 - 29 May 2014
|
Herausgeber:
|
Presutti, Valentina
|
Ort der Veröffentlichung:
|
Berlin [u.a.]
|
Verlag:
|
Springer
|
ISBN:
|
978-3-319-07442-9
|
ISSN:
|
0302-9743 , 1611-3349
|
Sprache der Veröffentlichung:
|
Englisch
|
Einrichtung:
|
Fakultät für Wirtschaftsinformatik und Wirtschaftsmathematik > Practical Computer Science II: Artificial Intelligence (Stuckenschmidt 2009-)
|
Fachgebiet:
|
004 Informatik
|
Abstract:
|
Open Information Extraction (OIE) systems like Nell and ReVerb have achieved impressive results by harvesting massive amounts of machine-readable knowledge with minimal supervision. However, the knowledge bases they produce still lack a clean, explicit semantic data model. This, on the other hand, could be provided by full-fledged semantic networks like DBpedia or Yago, which, in turn, could benefit from the additional coverage provided by Web-scale IE. In this paper, we bring these two strains of research together, and present a method to align terms from Nell with instances in DBpedia. Our approach is unsupervised in nature and relies on two key components. First, we automatically acquire probabilistic type information for Nell terms given a set of matching hypotheses. Second, we view the mapping task as the statistical inference problem of finding the most likely coherent mapping – i.e., the maximum a posteriori (MAP) mapping – based on the outcome of the first component used as soft constraint. These two steps are highly intertwined: accordingly, we propose an approach that iteratively refines type acquisition based on the output of the mapping generator, and vice versa. Experimental results on gold-standard data indicate that our approach outperforms a strong baseline, and is able to produce ever-improving mappings consistently across iterations.
|
Zusätzliche Informationen:
|
http://dx.doi.org/10.1007/978-3-319-07443-6_20
|
 | Dieser Eintrag ist Teil der Universitätsbibliographie. |
 | Das Dokument wird vom Publikationsserver der Universitätsbibliothek Mannheim bereitgestellt. |
Suche Autoren in
BASE:
Dutta, Arnab
;
Meilicke, Christian
;
Ponzetto, Simone Paolo
Google Scholar:
Dutta, Arnab
;
Meilicke, Christian
;
Ponzetto, Simone Paolo
ORCID:
Dutta, Arnab, Meilicke, Christian ORCID: https://orcid.org/0000-0002-0198-5396 and Ponzetto, Simone Paolo ORCID: https://orcid.org/0000-0001-7484-2049
Sie haben einen Fehler gefunden? Teilen Sie uns Ihren Korrekturwunsch bitte hier mit: E-Mail
Actions (login required)
 |
Eintrag anzeigen |
|
|