Automated Knowledge Base Extension Using Open Information
Dutta, Arnab
![[img]](https://madoc.bib.uni-mannheim.de/40469/1.hassmallThumbnailVersion/dutta.dissertation.pdf) 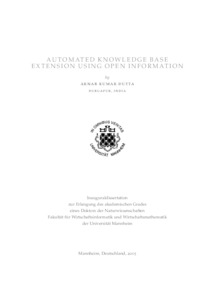 Vorschau |
|
PDF
dutta.dissertation.pdf
- Veröffentlichte Version
Download (1MB)
|
URL:
|
https://madoc.bib.uni-mannheim.de/40469
|
URN:
|
urn:nbn:de:bsz:180-madoc-404692
|
Dokumenttyp:
|
Dissertation
|
Erscheinungsjahr:
|
2016
|
Ort der Veröffentlichung:
|
Mannheim
|
Hochschule:
|
Universität Mannheim
|
Gutachter:
|
Stuckenschmidt, Heiner
|
Datum der mündl. Prüfung:
|
4 Februar 2016
|
Sprache der Veröffentlichung:
|
Englisch
|
Einrichtung:
|
Fakultät für Wirtschaftsinformatik und Wirtschaftsmathematik > Practical Computer Science II: Artificial Intelligence (Stuckenschmidt 2009-)
|
Fachgebiet:
|
004 Informatik
|
Normierte Schlagwörter (SWD):
|
Wissensverarbeitung , Wissenstechnik
|
Freie Schlagwörter (Englisch):
|
Data Integration , Markov Clustering , Enriching Knowledge Bases , Probabilistic Inference
|
Abstract:
|
Open Information Extractions (OIE) (like Nell, Reverb) frameworks provide us with domain independent facts in natural language forms containing knowledge from varied sources. Extraction mechanisms for structured knowledge bases (KB) (like DBpedia, Yago) often fail to retrieve such facts due to its resource specific extraction schemes. Hence, the structured KBs can extend themselves by augmenting
their coverage with the facts discovered by OIE systems. This possibility motivates us to integrate these two genres of extractions into one interactive framework. In this work, we present a complete, ontology independent, generalized architecture for achieving this integration. Our proposed solution is modularized which solves a specific set of tasks: (1) mapping subject and object terms from OIE
facts to KB instances (2) mapping the OIE relational phrases to object properties defined in the KB. Furthermore, in an open extraction setting identical semantic relationships can be represented by different surface forms, making it necessary to group them together. To solve this problem, (3) we propose the use of markov clustering to cluster OIE relations. Key to our approach lies in exploiting the inherent dependancies between relations and its arguments. This makes our approach completely context agnostic and generally applicable. We evaluated our method on the two state of the art extraction systems, achieving over 85% precision on instance mappings and over 90% for the relation mappings. We also created a
distant supervision based gold standard for the purpose and the data has been released as part of this work. Furthermore, we analyze the effect of clustering and empirically show its effectiveness as a relation mapping technique over other techniques. Overall, our work positions itself on the intersection of information extraction, ontology mapping and reasoning.
|
 | Dieser Eintrag ist Teil der Universitätsbibliographie. |
 | Das Dokument wird vom Publikationsserver der Universitätsbibliothek Mannheim bereitgestellt. |
Suche Autoren in
Sie haben einen Fehler gefunden? Teilen Sie uns Ihren Korrekturwunsch bitte hier mit: E-Mail
Actions (login required)
 |
Eintrag anzeigen |
|
|