On Leveraging Statistical and Relational Information for the Representation and Recognition of Complex Human Activities
Helaoui, Rim
![[img]](https://madoc.bib.uni-mannheim.de/40557/1.hassmallThumbnailVersion/RimHelaoui_Dissertation_PublishedVersion.pdf) 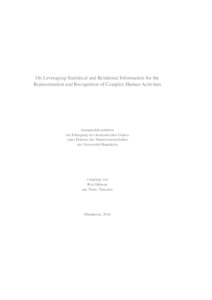 Vorschau |
|
PDF
RimHelaoui_Dissertation_PublishedVersion.pdf
- Veröffentlichte Version
Download (3MB)
|
URL:
|
https://madoc.bib.uni-mannheim.de/40557
|
URN:
|
urn:nbn:de:bsz:180-madoc-405578
|
Dokumenttyp:
|
Dissertation
|
Erscheinungsjahr:
|
2016
|
Ort der Veröffentlichung:
|
Mannheim, Germany
|
Hochschule:
|
Universität Mannheim
|
Gutachter:
|
Stuckenschmidt, Heiner
|
Datum der mündl. Prüfung:
|
19 Februar 2016
|
Sprache der Veröffentlichung:
|
Englisch
|
Einrichtung:
|
Fakultät für Wirtschaftsinformatik und Wirtschaftsmathematik > Practical Computer Science II: Artificial Intelligence (Stuckenschmidt 2009-)
|
Fachgebiet:
|
004 Informatik
|
Normierte Schlagwörter (SWD):
|
Aktivität , Erkenntnis
|
Freie Schlagwörter (Englisch):
|
Activity recognition , statistical relational approach , Markov logic , log-linear description logics , probabilistic graphical models , first-order logic , ontology , fine-grained activity recognition , multi-level activity recognition , hybrid approach
|
Abstract:
|
Machine activity recognition aims to automatically predict human activities from a series of sensor signals. It is a key aspect to several emerging applications, especially in the pervasive computing field. However, this problem faces several challenges due to the complex, relational and ambiguous nature of human activities.
These challenges still defy the majority of traditional pattern recognition approaches, whether they are knowledge-based or data-driven. Concretely, the current approaches to activity recognition in sensor environments fall short to represent, reason or learn under uncertainty, complex relational structure, rich temporal context and abundant common-sense knowledge. Motivated by these shortcomings, our work focuses on the combination of both data-driven and knowledge-based
paradigms in order to address this problem. In particular, we propose two logic-based statistical relational activity recognition frameworks which we describe in two different parts.
The first part presents a Markov logic-based framework addressing the recognition of complex human activities under realistic settings. Markov logic is a highly flexible statistical relational formalism combining the power of first-order logic with Markov networks by attaching real-valued weights to formulas in first-order logic. Thus, it unites both symbolic and probabilistic reasoning and allows to model the complex relational structure as well as the inherent uncertainty underlying human activities and sensor data. We focus on addressing the challenge of recognizing interleaved and concurrent activities while preserving the intuitiveness and flexibility of the modelling task. Using three different models we evaluate and prove the viability of using Markov logic networks for that problem statement. We also demonstrate the crucial impact of domain knowledge on the recognition outcome.
Implementing an exhaustive model including heterogeneous information sources comes, however, at considerable knowledge engineering efforts. Hence, employing a standard, widely used formalism can alleviate that by enhancing the portability, the re-usability and the extension of the model. In the second part of this document, we apply a hybrid approach that goes one step further than Markov logic network towards a formal, yet intuitive conceptualization of the domain of discourse. Concretely, we propose an activity recognition framework based on log-linear description logic, a probabilistic variant of description logics.
Log-linear description logic leverages the principles of Markov logic while allowing for a formal conceptualization of the domain of discourse, backed up with powerful reasoning and consistency check tools. Based on principles from the activity theory, we focus on addressing the challenge of representing and recognizing human activities at three levels of granularity: operations, actions and activities. Complying with real-life scenarios, we assess and discuss the viability of the proposed framework. In particular, we show the positive impact of augmenting
the proposed multi-level activity ontology with weights compared to using its conventional weight-free variant.
|
 | Dieser Eintrag ist Teil der Universitätsbibliographie. |
 | Das Dokument wird vom Publikationsserver der Universitätsbibliothek Mannheim bereitgestellt. |
Suche Autoren in
Sie haben einen Fehler gefunden? Teilen Sie uns Ihren Korrekturwunsch bitte hier mit: E-Mail
Actions (login required)
 |
Eintrag anzeigen |
|