Processing tree models for discrete and continuous variables
Heck, Daniel W.
![[img]](https://madoc.bib.uni-mannheim.de/42815/1.hassmallThumbnailVersion/heck_dissertation.pdf) 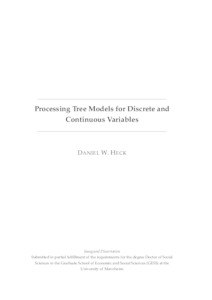 Vorschau |
|
PDF
heck_dissertation.pdf
- Veröffentlichte Version
Download (6MB)
|
URL:
|
https://ub-madoc.bib.uni-mannheim.de/42815
|
URN:
|
urn:nbn:de:bsz:180-madoc-428151
|
Dokumenttyp:
|
Dissertation
|
Erscheinungsjahr:
|
2017
|
Ort der Veröffentlichung:
|
Mannheim
|
Hochschule:
|
Universität Mannheim
|
Gutachter:
|
Erdfelder, Edgar
|
Datum der mündl. Prüfung:
|
5 September 2017
|
Sprache der Veröffentlichung:
|
Englisch
|
Einrichtung:
|
Fakultät für Sozialwissenschaften > Kognitive Psychologie u. Differentielle Psychologie (Erdfelder 2002-2019) Außerfakultäre Einrichtungen > GESS - CDSS (SOWI)
|
Fachgebiet:
|
150 Psychologie
|
Normierte Schlagwörter (SWD):
|
Mathematische Psychologie , Modellierung , Kognitiver Prozess
|
Freie Schlagwörter (Englisch):
|
Cognitive modeling , multinomial processing tree models , decision making , mathematical psychology
|
Abstract:
|
In psychology, multinomial processing tree (MPT) models explain how qualitatively different processes determine observed response behavior. Even though they have successfully been used in many applications, MPT models are inherently limited to discrete data such as choice frequencies. In my thesis, I therefore propose two new approaches that extend MPT models to continuous variables such as response times (RTs), fine-grained response scales, or process-tracing measures. Both approaches assume that continuous variables follow a finite mixture distribution with mixture weights determined by the processing tree structure and state-specific, continuous component distributions.
In the first approach, RT-extended versions of MPT models are obtained by categorizing continuous observations into a finite number of intervals. Thereby, the RT component distributions can be estimated by histograms, allowing researchers to test the relative speed of different latent processes as demonstrated for the two-high-threshold model of recognition memory. In a theoretical paper, the new method is used to develop an RT-extended MPT model of recognition-based decisions and test competing process models. Even though the two theoretical accounts under consideration differ strongly and assume serial and parallel information integration, respectively, empirical tests have proved to be difficult without the new measurement model.
As a second approach, I developed the class of generalized processing tree (GPT) models that assume parametric families instead of histograms for the continuous component distributions. The main advantages of GPT models are that they reduce the flexibility of the component distributions, can result in more precise estimates for the processing-tree parameters compared to MPT models, and allow for modeling one or more continuous variables jointly. In a first empirical application, a GPT model provided a good account of mouse-tracking data in a semantic-categorization paradigm. In sum, my thesis lays the foundations for new empirical tests of discrete-state theories of cognition by jointly modeling discrete and continuous variables.
|
 | Dieser Eintrag ist Teil der Universitätsbibliographie. |
 | Das Dokument wird vom Publikationsserver der Universitätsbibliothek Mannheim bereitgestellt. |
Suche Autoren in
Sie haben einen Fehler gefunden? Teilen Sie uns Ihren Korrekturwunsch bitte hier mit: E-Mail
Actions (login required)
 |
Eintrag anzeigen |
|
|