Extending cross-domain knowledge bases with long tail entities using web table data
Oulabi, Yaser
;
Bizer, Christian
![[img]](https://madoc.bib.uni-mannheim.de/49189/1.hassmallThumbnailVersion/EDBT19_paper_157.pdf) 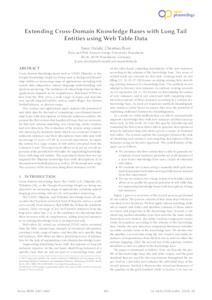 Vorschau |
|
PDF
EDBT19_paper_157.pdf
- Veröffentlichte Version
Download (2MB)
|
DOI:
|
https://doi.org/10.5441/002/edbt.2019.34
|
URL:
|
https://ub-madoc.bib.uni-mannheim.de/49189
|
Weitere URL:
|
http://openproceedings.org/2019/conf/edbt/EDBT19_p...
|
URN:
|
urn:nbn:de:bsz:180-madoc-491897
|
Dokumenttyp:
|
Konferenzveröffentlichung
|
Erscheinungsjahr:
|
2019
|
Buchtitel:
|
Advances in Database Technology - 22nd International Conference on Extending Database Technology, EDBT 2019, Lisbon, Portugal, March 26-29, 2019 : proceedings
|
Seitenbereich:
|
385-396
|
Veranstaltungstitel:
|
22. EDBT 2019
|
Veranstaltungsort:
|
Lisbon, Portugal
|
Veranstaltungsdatum:
|
March 26-29, 2019
|
Herausgeber:
|
Herschel, Melanie
|
Ort der Veröffentlichung:
|
Konstanz
|
Verlag:
|
OpenProceedings.org
|
ISBN:
|
978-3-89318-081-3
|
ISSN:
|
2367-2005
|
Verwandte URLs:
|
|
Sprache der Veröffentlichung:
|
Englisch
|
Einrichtung:
|
Fakultät für Wirtschaftsinformatik und Wirtschaftsmathematik > Information Systems V: Web-based Systems (Bizer 2012-)
|
Lizenz:
|
Creative Commons Namensnennung 4.0 International (CC BY 4.0)
|
Fachgebiet:
|
004 Informatik
|
Freie Schlagwörter (Englisch):
|
Knowledge Base Completion , Set Completion , Slot Filling , Long Tail Entities , Web Tables , Knowledge Graphs
|
Abstract:
|
Cross-domain knowledge bases such as YAGO, DBpedia, or the Google Knowledge Graph are being used as background knowledge within an increasing range of applications including web search, data integration, natural language understanding, and question answering. The usefulness of a knowledge base for these applications depends on its completeness. Relational HTML tables from the Web cover a wide range of topics and describe very specific long tail entities, such as small villages, less-known football players, or obscure songs.
This systems and applications paper explores the potential of web table data for the task of completing cross-domain knowledge bases with descriptions of formerly unknown entities. We present the first system that handles all steps that are necessary for this task: schema matching, row clustering, entity creation, and new detection. The evaluation of the system using a manually labeled gold standard shows that it can construct formerly unknown instances and their descriptions from table data with an average F1 score of 0.80. In a second experiment, we apply the system to a large corpus of web tables extracted from the Common Crawl. This experiment allows us to get an overall impression of the potential of web tables for augmenting knowledge bases with long tail entities. The experiment shows that we can augment the DBpedia knowledge base with descriptions of 14 thousand new football players as well as 187 thousand new songs. The accuracy of the facts describing these instances is 0.90.
|
Zusätzliche Informationen:
|
Online-Ressource
|
 | Dieser Eintrag ist Teil der Universitätsbibliographie. |
 | Das Dokument wird vom Publikationsserver der Universitätsbibliothek Mannheim bereitgestellt. |
Suche Autoren in
Sie haben einen Fehler gefunden? Teilen Sie uns Ihren Korrekturwunsch bitte hier mit: E-Mail
Actions (login required)
 |
Eintrag anzeigen |
|
|