Data-driven decision support for perishable goods
Huber, Jakob
![[img]](https://madoc.bib.uni-mannheim.de/52366/1.hassmallThumbnailVersion/Jakob-Huber_PhD-Thesis-2019.pdf) 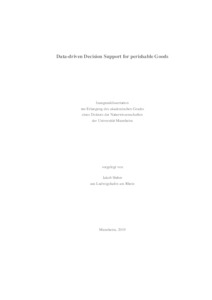 Vorschau |
|
PDF
Jakob-Huber_PhD-Thesis-2019.pdf
- Veröffentlichte Version
Download (1MB)
|
URL:
|
https://madoc.bib.uni-mannheim.de/52366
|
URN:
|
urn:nbn:de:bsz:180-madoc-523663
|
Dokumenttyp:
|
Dissertation
|
Erscheinungsjahr:
|
2019
|
Ort der Veröffentlichung:
|
Mannheim
|
Hochschule:
|
Universität Mannheim
|
Gutachter:
|
Stuckenschmidt, Heiner
|
Datum der mündl. Prüfung:
|
27 September 2019
|
Sprache der Veröffentlichung:
|
Englisch
|
Einrichtung:
|
Fakultät für Wirtschaftsinformatik und Wirtschaftsmathematik > Practical Computer Science II: Artificial Intelligence (Stuckenschmidt 2009-)
|
Fachgebiet:
|
004 Informatik
|
Normierte Schlagwörter (SWD):
|
Datenanalyse , Prognose , Entscheidungsunterstützung , Maschinelles Lernen , Künstliche Intelligenz , Einzelhandel , Bestandsmanagement
|
Freie Schlagwörter (Englisch):
|
decision support , predictive analytics , prescriptive analytics , machine learning , forecasting , inventory management , retailing
|
Abstract:
|
Retailers offering perishable consumer goods such as baked goods have to make hundreds of ordering decisions every day because they typically operate numerous stores and offer a wide range of products. Daily decisions or even intraday decisions are necessary as perishable goods deteriorate quickly and can usually only be sold on one day. Obviously, decision making concerning ordering quantities is a challenging but important task for each retailer as it affects its operational performance. Ordering too little leads to unsatisfied customers while ordering too much leads to discarded goods, which is a major cost factor. In practice, store managers are typically responsible for decisions related to perishable goods, which is not optimal for various reasons. Most importantly, the task is time consuming and some store managers may not have the necessary skills, which results in poor decisions. Hence, our goal is to develop and evaluate methods to support the decision-making process, which is made possible by advances in information technology and data analysis. In particular, we investigate how to exploit large datasets to make better decisions.
For daily ordering decisions, we prose data-driven solution approaches for inventory management models that capture the trade-off of ordering too much or ordering too little such that the profits are maximized. First, we optimize the order quantity for each product independently. Second, we consider demand substitution and jointly optimize the order quantities of substitutable products. For intraday decisions, we formulate a scheduling problem for the optimization of baking plans based on hourly forecasts.
Demand forecasts are an essential input for operational decisions. However, retail forecasting research is mainly devoted to weekly data using statistical time series models or linear regression models, whereas large-scale forecasting on daily data is understudied. We phrase the forecasting problem as a supervised Machine Learning task and conduct a comprehensive empirical evaluation to illustrate the suitability of Machine Learning methods.
We empirically evaluate our solution approaches on real-world datasets from the bakery domain that are enriched with explanatory feature data. We find that our approaches perform competitive to state-of-the-art methods. Data-driven approaches substantially outperform traditional methods if the dataset is large enough. We also find that the benefit of improved forecasting dominates other potential benefits of data-driven solution methods for decision optimization. Overall, we conclude that data-driven decision support for perishable goods is feasible and superior to alternatives that are based on unreasonable assumptions or established time series models.
|
 | Dieser Eintrag ist Teil der Universitätsbibliographie. |
 | Das Dokument wird vom Publikationsserver der Universitätsbibliothek Mannheim bereitgestellt. |
Suche Autoren in
Sie haben einen Fehler gefunden? Teilen Sie uns Ihren Korrekturwunsch bitte hier mit: E-Mail
Actions (login required)
 |
Eintrag anzeigen |
|
|