You CAN teach an old dog new tricks! On training knowledge graph embeddings
Ruffinelli, Daniel
;
Broscheit, Samuel
;
Gemulla, Rainer
![[img]](https://madoc.bib.uni-mannheim.de/54954/1.hassmallThumbnailVersion/you_can_teach_an_old_dog_new_tricks_on_training_knowledge_graph_embeddings.pdf) 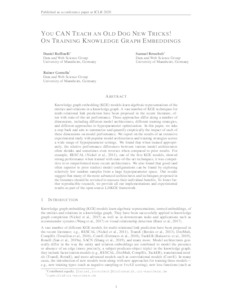 Vorschau |
|
PDF
you_can_teach_an_old_dog_new_tricks_on_training_knowledge_graph_embeddings.pdf
- Veröffentlichte Version
Download (428kB)
|
URL:
|
https://madoc.bib.uni-mannheim.de/54954
|
Weitere URL:
|
https://openreview.net/forum?id=BkxSmlBFvr
|
URN:
|
urn:nbn:de:bsz:180-madoc-549543
|
Dokumenttyp:
|
Konferenzveröffentlichung
|
Erscheinungsjahr:
|
2020
|
Buchtitel:
|
ICLR 2020 : Eighth International Conference on Learning Representations : virtual conference, formerly Addis Ababa ETHIOPIA, Sun Apr 26th through May 1st
|
Seitenbereich:
|
1-12
|
Veranstaltungstitel:
|
ICLR 2020
|
Veranstaltungsort:
|
Online
|
Veranstaltungsdatum:
|
26.4.-1.5.2020
|
Herausgeber:
|
Rush, Alexander
|
Ort der Veröffentlichung:
|
La Jolla, CA
|
Verlag:
|
ICLR
|
Sprache der Veröffentlichung:
|
Englisch
|
Einrichtung:
|
Fakultät für Wirtschaftsinformatik und Wirtschaftsmathematik > Practical Computer Science I: Data Analytics (Gemulla 2014-)
|
Lizenz:
|
Creative Commons Namensnennung 4.0 International (CC BY 4.0)
|
Fachgebiet:
|
004 Informatik
|
Freie Schlagwörter (Englisch):
|
knowledge graph embeddings , hyperparameter optimization
|
Abstract:
|
Knowledge graph embedding (KGE) models learn algebraic representations of the entities and relations in a knowledge graph. A vast number of KGE techniques for multi-relational link prediction have been proposed in the recent literature, often with state-of-the-art performance. These approaches differ along a number of dimensions, including different model architectures, different training strategies, and different approaches to hyperparameter optimization. In this paper, we take a step back and aim to summarize and quantify empirically the impact of each of these dimensions on model performance. We report on the results of an extensive experimental study with popular model architectures and training strategies across a wide range of hyperparameter settings. We found that when trained appropriately, the relative performance differences between various model architectures often shrinks and sometimes even reverses when compared to prior results. For example, RESCAL~\citep{nickel2011three}, one of the first KGE models, showed strong performance when trained with state-of-the-art techniques; it was competitive to or outperformed more recent architectures. We also found that good (and often superior to prior studies) model configurations can be found by exploring relatively few random samples from a large hyperparameter space. Our results suggest that many of the more advanced architectures and techniques proposed in the literature should be revisited to reassess their individual benefits. To foster further reproducible research, we provide all our implementations and experimental results as part of the open source LibKGE framework.
|
 | Dieser Eintrag ist Teil der Universitätsbibliographie. |
 | Das Dokument wird vom Publikationsserver der Universitätsbibliothek Mannheim bereitgestellt. |
Suche Autoren in
Sie haben einen Fehler gefunden? Teilen Sie uns Ihren Korrekturwunsch bitte hier mit: E-Mail
Actions (login required)
 |
Eintrag anzeigen |
|
|