Combining fairness and causal graphs to advance both
Cohausz, Lea
;
Kappenberger, Jakob
;
Stuckenschmidt, Heiner
URN:
|
urn:nbn:de:bsz:180-madoc-683119
|
Dokumenttyp:
|
Konferenzveröffentlichung
|
Erscheinungsjahr:
|
2024
|
Buchtitel:
|
Fairness and Bias in AI : Proceedings of the 2nd Workshop on Fairness and Bias in AI, co-located with 27th European Conference on Artificial Intelligence (ECAI 2024)
|
Titel einer Zeitschrift oder einer Reihe:
|
CEUR Workshop Proceedings
|
Band/Volume:
|
3808
|
Seitenbereich:
|
1-14
|
Veranstaltungstitel:
|
AEQUITAS 2024
|
Veranstaltungsdatum:
|
20. Oktober 2024
|
Herausgeber:
|
Calegari, Roberta
;
Dignum, Virginia
;
O'Sullivan, Barry
|
Ort der Veröffentlichung:
|
Aachen, Germany
|
Verlag:
|
RWTH Aachen
|
ISSN:
|
1613-0073
|
Verwandte URLs:
|
|
Sprache der Veröffentlichung:
|
Englisch
|
Einrichtung:
|
Fakultät für Wirtschaftsinformatik und Wirtschaftsmathematik > Practical Computer Science II: Artificial Intelligence (Stuckenschmidt 2009-)
|
Bereits vorhandene Lizenz:
|
Creative Commons Namensnennung 4.0 International (CC BY 4.0)
|
Fachgebiet:
|
004 Informatik
|
Abstract:
|
Recent work on fairness in Machine Learning (ML) demonstrated that it is important to know the causal relationships among variables to decide whether a sensitive variable may have a problematic influence on the prediction and what fairness metric and potential bias mitigation strategy to use. These causal relationships can best be represented by Directed Acyclic Graphs (DAGs). However, so far, there is no clear classification of different causal structures containing sensitive variables in these DAGs. This paper’s first contribution is classifying the structures into four classes, each with different implications for fairness. However, we first need to learn the DAGs to uncover these structures. Structure learning algorithms exist but currently do not make systematic use of the background knowledge we have when considering fairness in ML, although the background knowledge could increase the correctness of the DAGs. Therefore, the second contribution is an adaptation of the structure learning methods. This is evaluated in the paper, demonstrating that the adaptation increases correctness. The two contributions of this paper are implemented in our publicly available Python package causalfair, allowing everyone to evaluate which relationships in the data might become problematic when applying ML.
|
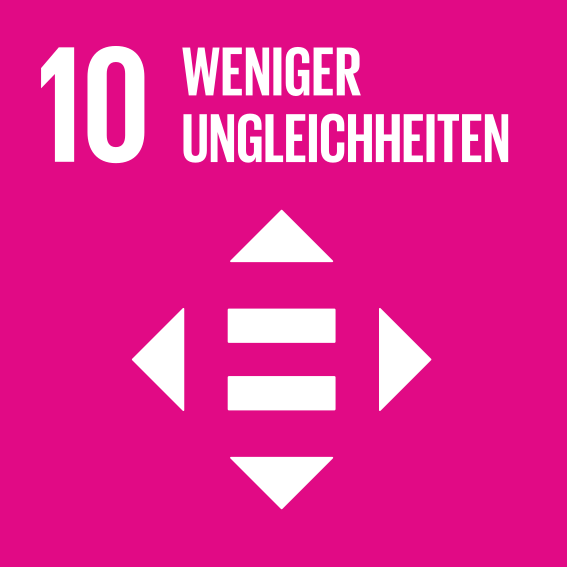
 | Dieser Eintrag ist Teil der Universitätsbibliographie. |
 | Das Dokument wird vom Publikationsserver der Universitätsbibliothek Mannheim bereitgestellt. |
Suche Autoren in
Sie haben einen Fehler gefunden? Teilen Sie uns Ihren Korrekturwunsch bitte hier mit: E-Mail
Actions (login required)
 |
Eintrag anzeigen |
|
|