Investigating demographic features and their connection to performance, predictions, and fairness in EDM models
Cohausz, Lea
;
Tschalzev, Andrej
;
Bartelt, Christian
;
Stuckenschmidt, Heiner

DOI:
|
https://doi.org/10.5281/zenodo.14503515
|
URL:
|
https://jedm.educationaldatamining.org/index.php/J...
|
Dokumenttyp:
|
Zeitschriftenartikel
|
Erscheinungsjahr:
|
2024
|
Titel einer Zeitschrift oder einer Reihe:
|
Journal of Educational Data Mining
|
Band/Volume:
|
16
|
Heft/Issue:
|
2
|
Seitenbereich:
|
177-213
|
Ort der Veröffentlichung:
|
Montreal
|
Verlag:
|
International Educational Data Mining Society
|
ISSN:
|
2157-2100
|
Sprache der Veröffentlichung:
|
Englisch
|
Einrichtung:
|
Fakultät für Wirtschaftsinformatik und Wirtschaftsmathematik > Practical Computer Science II: Artificial Intelligence (Stuckenschmidt 2009-)
|
Fachgebiet:
|
004 Informatik
|
Abstract:
|
Although using demographic features for predictive models in Educational Data Mining (EDM) has to be considered very problematic from a fairness point of view and is currently critically discussed in the field, they are, in practice, frequently used without much deliberate thought. Their use and the discussion around their use mostly rely on the belief that they help achieve high model performance. In this paper, we theoretically and empirically assess the mechanisms that make them relevant for prediction and what this means for notions of fairness. Using four datasets for at-risk prediction, we find evidence that re- moving demographic features does not usually lead to a decrease in performance but also that we may sometimes be wrong in aiming to achieve the most accurate predictions. Furthermore, we show that mod- els, nonetheless, place weight on these features when they are included – highlighting the need to exclude them. Additionally, we show that even when demographic features are excluded, some fairness concerns relating to group fairness metrics may persist. These findings strongly highlight the need to know more about the causal mechanisms underlying the data and to think critically about demographic features in each specific setting – emphasizing the need for more research on how demographic features influence educational attainment. Our code is available at: https://github.com/atschalz/edm.
|
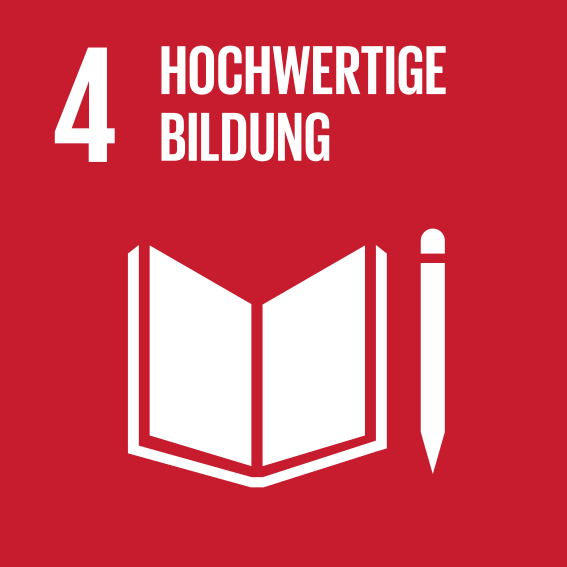 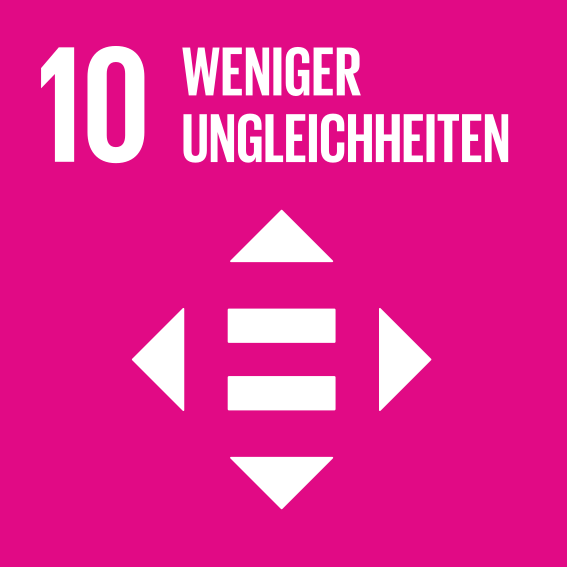
 | Dieser Eintrag ist Teil der Universitätsbibliographie. |
Suche Autoren in
BASE:
Cohausz, Lea
;
Tschalzev, Andrej
;
Bartelt, Christian
;
Stuckenschmidt, Heiner
Google Scholar:
Cohausz, Lea
;
Tschalzev, Andrej
;
Bartelt, Christian
;
Stuckenschmidt, Heiner
ORCID:
Cohausz, Lea ; Tschalzev, Andrej ORCID: 0000-0002-0638-5744 ; Bartelt, Christian ; Stuckenschmidt, Heiner ORCID: 0000-0002-0209-3859
Sie haben einen Fehler gefunden? Teilen Sie uns Ihren Korrekturwunsch bitte hier mit: E-Mail
Actions (login required)
 |
Eintrag anzeigen |
|
|