Bivariate Gaussian random fields : models, simulation, and inference
Moreva, Olga
![[img]](https://madoc.bib.uni-mannheim.de/45380/1.hassmallThumbnailVersion/DissertationOlgaMoreva.pdf) 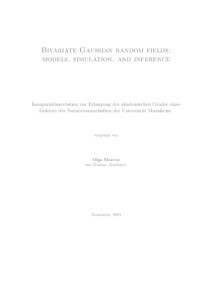 Vorschau |
|
PDF
DissertationOlgaMoreva.pdf
- Veröffentlichte Version
Download (5MB)
|
URL:
|
https://ub-madoc.bib.uni-mannheim.de/45380
|
URN:
|
urn:nbn:de:bsz:180-madoc-453800
|
Dokumenttyp:
|
Dissertation
|
Erscheinungsjahr:
|
2018
|
Ort der Veröffentlichung:
|
Mannheim
|
Hochschule:
|
Universität Mannheim
|
Gutachter:
|
Schlather, Martin
|
Datum der mündl. Prüfung:
|
22 Juni 2018
|
Sprache der Veröffentlichung:
|
Englisch
|
Einrichtung:
|
Fakultät für Wirtschaftsinformatik und Wirtschaftsmathematik > Applied Stochastics (Schlather 2012-)
|
Fachgebiet:
|
510 Mathematik
|
Normierte Schlagwörter (SWD):
|
Geostatistik , Kovarianzfunktion , Positiv-definite Funktion
|
Freie Schlagwörter (Englisch):
|
circulant embedding , cokriging , compactly supported covariance function , cut-off embedding , multivariate covariance function , multivariate Gaussian random field , multivariate geostatistics
|
Abstract:
|
Spatial data with several components, such as observations of air temperature
and pressure in a certain geographical region or the content of two metals in a
geological deposit, require models which can capture the spatial dependence
structure of individual components and the relationship between them. In a
wealth of applications, multivariate Gaussian random fields are sensible models
for multivariate spatial data and their second order structure specifies the
marginal correlations and the cross-correlations between the components. In this
thesis we focus on covariance models and simulation techniques for bivariate fields.
In Chapter 2 we summarize some definitions and facts from univariate and
multivariate Geostatistics which are essential for the subsequent chapters.
Chapter 3 introduces two novel bivariate parametric covariance models, the
powered exponential (or stable) covariance model and the generalized Cauchy
covariance model. Both models allow for flexible smoothness, variance, scale,
and cross-correlation parameters. The smoothness parameter is in (0, 1].
Additionally, the bivariate generalized Cauchy model allows for distinct long
range parameters. The results are based on general sufficient conditions for the
positive definiteness of 2×2-matrix valued functions. These conditions are easy
to check, since they require only computing the derivatives of a bivariate
covariance function and calculating an infimum of a function of one variable. We
also show that the univariate spherical model can be generalized to the
bivariate case with spherical marginal and cross-covariance functions only in a
trivial way.
Circulant embedding is a powerful algorithm for fast simulation of stationary
Gaussian random fields on a rectangular grid in R^n , which works perfectly for
compactly supported covariance functions. Cut-off circulant embedding techniques
have been developed for univariate random fields for dimensions up to R^3 and
rely on the modification of a covariance function outside the simulation window,
such that the modified covariance function is compactly supported. In Chapter 4
we propose extensions of the cut-off approach for bivariate Gaussian random
fields. In particular, we provide a method for simulating bivariate fields with
a bivariate powered exponential covariance model and the full bivariate Matérn
covariance model for certain sets of parameters. On the way we extend the
cut-off circulant embedding method even for univariate models.
In Chapter 5 we illustrate the use of the bivariate powered exponential model
for a data
example.
|
 | Dieser Eintrag ist Teil der Universitätsbibliographie. |
 | Das Dokument wird vom Publikationsserver der Universitätsbibliothek Mannheim bereitgestellt. |
Suche Autoren in
Sie haben einen Fehler gefunden? Teilen Sie uns Ihren Korrekturwunsch bitte hier mit: E-Mail
Actions (login required)
 |
Eintrag anzeigen |
|
|