Integrating predictive and prescriptive
analytics for assortment optimization - a machine learning-based approach
Sadeghi, Niloufar
;
Khayyati, Siamak
;
Schön, Cornelia

Dokumenttyp:
|
Präsentation auf Konferenz
|
Erscheinungsjahr:
|
2024
|
Veranstaltungstitel:
|
EURO 2024, 33rd European Conference on Operational Research
|
Veranstaltungsort:
|
Copenhagen, Denmark
|
Veranstaltungsdatum:
|
30.06.-03.07.2024
|
Verwandte URLs:
|
|
Sprache der Veröffentlichung:
|
Englisch
|
Einrichtung:
|
Fakultät für Betriebswirtschaftslehre > Service Operations Management (Schön 2014-)
|
Fachgebiet:
|
650 Management
|
Abstract:
|
Product line design is one of the key problems that firms need to solve. Therefore, they employ techniques to predict customer choice behaviour and optimize the performance of the product assortment they aim to offer.In most cases, the prediction problem and the optimization problem are defined as separate problems and solved in a sequential manner where first, the choice model is estimated and second, the assortment optimization problem is solved, using the choice model parameters as an input. Integrating estimation and optimization provides an opportunity for the empirical model to be more accurate where it matters – close to the optimal assortment. We develop a MILP formulation that is able to solve the two problems jointly. Using numerical experiments, we analyse under what conditions and to which extent the integrated approach is superior to the sequential approach.
Keywords
Revenue Management and Pricing
|
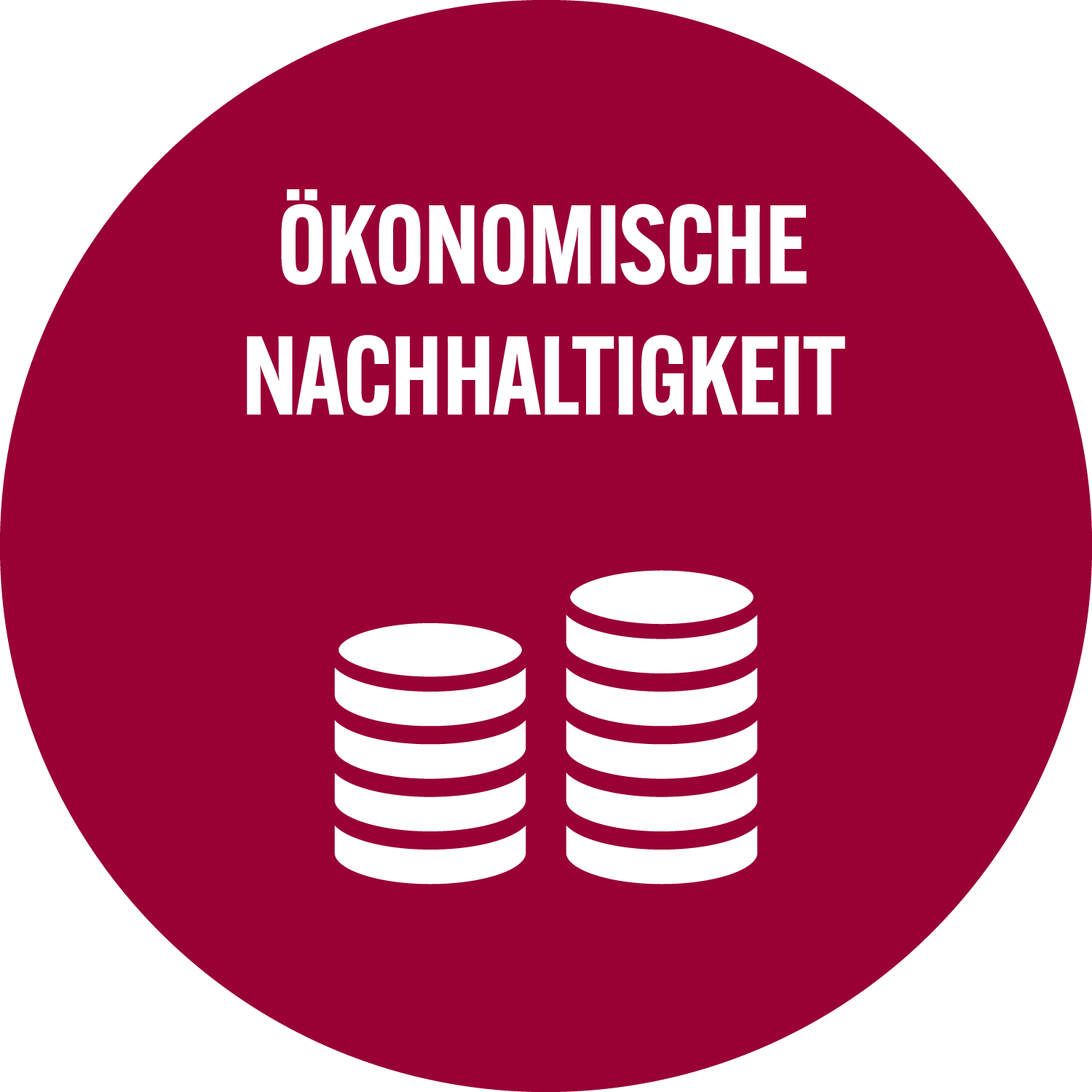
 | Dieser Eintrag ist Teil der Universitätsbibliographie. |
Suche Autoren in
Sie haben einen Fehler gefunden? Teilen Sie uns Ihren Korrekturwunsch bitte hier mit: E-Mail
Actions (login required)
 |
Eintrag anzeigen |
|
|