A unified framework for frequent sequence mining with subsequence constraints
Beedkar, Kaustubh
;
Gemulla, Rainer
;
Mertens, Wim
![[img]](https://madoc.bib.uni-mannheim.de/48227/1.hassmallThumbnailVersion/A%20Unified%20Framework%20for%20Frequent%20Sequence%20Mining%20with%20Subsequence%20Constraints.pdf) 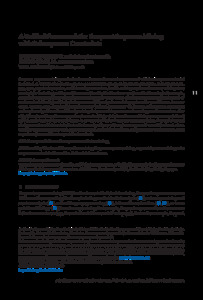 Vorschau |
|
PDF
A Unified Framework for Frequent Sequence Mining with Subsequence Constraints.pdf
- Veröffentlichte Version
Download (1MB)
|
DOI:
|
https://doi.org/10.1145/3321486
|
URL:
|
https://madoc.bib.uni-mannheim.de/48227
|
Weitere URL:
|
https://dl.acm.org/citation.cfm?id=3321486
|
URN:
|
urn:nbn:de:bsz:180-madoc-482279
|
Dokumenttyp:
|
Zeitschriftenartikel
|
Erscheinungsjahr:
|
2019
|
Titel einer Zeitschrift oder einer Reihe:
|
ACM Transactions on Database Systems : TODS
|
Band/Volume:
|
44
|
Heft/Issue:
|
3
|
Seitenbereich:
|
11:1-11:42
|
Ort der Veröffentlichung:
|
New York, NY
|
Verlag:
|
ACM Press
|
ISSN:
|
0362-5915 , 1557-4644
|
Sprache der Veröffentlichung:
|
Englisch
|
Einrichtung:
|
Fakultät für Wirtschaftsinformatik und Wirtschaftsmathematik > Practical Computer Science I: Data Analytics (Gemulla 2014-)
|
Lizenz:
|
Creative Commons Namensnennung 4.0 International (CC BY 4.0)
|
Fachgebiet:
|
004 Informatik
|
Abstract:
|
Frequent sequence mining methods often make use of constraints to control which subsequences should be mined. A variety of such subsequence constraints has been studied in the literature, including length, gap, span, regular-expression, and hierarchy constraints. In this article, we show that many subsequence constraints—including and beyond those considered in the literature—can be unified in a single framework. A unified treatment allows researchers to study jointly many types of subsequence constraints (instead of each one individually) and helps to improve usability of pattern mining systems for practitioners. In more detail, we propose a set of simple and intuitive “pattern expressions” to describe subsequence constraints and explore algorithms for efficiently mining frequent subsequences under such general constraints. Our algorithms translate pattern expressions to succinct finite-state transducers, which we use as computational model, and simulate these transducers in a way suitable for frequent sequence mining. Our experimental study on real-world datasets indicates that our algorithms—although more general—are efficient and, when used for sequence mining with prior constraints studied in literature, competitive to (and in some cases superior to) state-of-the-art specialized methods.
|
 | Dieser Eintrag ist Teil der Universitätsbibliographie. |
 | Das Dokument wird vom Publikationsserver der Universitätsbibliothek Mannheim bereitgestellt. |
Suche Autoren in
Sie haben einen Fehler gefunden? Teilen Sie uns Ihren Korrekturwunsch bitte hier mit: E-Mail
Actions (login required)
 |
Eintrag anzeigen |
|
|