Using weak supervision to identify long-tail entities for knowledge base completion
Oulabi, Yaser
;
Bizer, Christian
![[img]](https://madoc.bib.uni-mannheim.de/52759/1.hassmallThumbnailVersion/OulabiBizer2019weaksupervision.pdf) 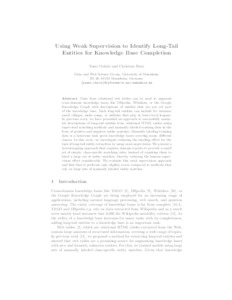 Vorschau |
|
PDF
OulabiBizer2019weaksupervision.pdf
- Veröffentlichte Version
Download (439kB)
|
DOI:
|
https://doi.org/10.1007/978-3-030-33220-4_7
|
URL:
|
https://madoc.bib.uni-mannheim.de/52759
|
Weitere URL:
|
https://link.springer.com/chapter/10.1007%2F978-3-...
|
URN:
|
urn:nbn:de:bsz:180-madoc-527597
|
Dokumenttyp:
|
Konferenzveröffentlichung
|
Erscheinungsjahr:
|
2019
|
Buchtitel:
|
Semantic systems : The power of AI and knowledge graphs : 15th International Conference, SEMANTiCS 2019, Karlsruhe, Germany, September 9-12, 2019, proceedings
|
Titel einer Zeitschrift oder einer Reihe:
|
Lecture Notes in Computer Science
|
Band/Volume:
|
11702
|
Seitenbereich:
|
83-98
|
Veranstaltungstitel:
|
SEMANTiCS 2019
|
Veranstaltungsort:
|
Karlsruhe, Germany
|
Veranstaltungsdatum:
|
Sept. 09-12, 2019
|
Herausgeber:
|
Acosta, Maribel
|
Ort der Veröffentlichung:
|
Berlin [u.a.]
|
Verlag:
|
Springer
|
ISBN:
|
978-3-030-33219-8 , 978-3-030-33220-4
|
ISSN:
|
0302-9743 , 1611-3349
|
Sprache der Veröffentlichung:
|
Englisch
|
Einrichtung:
|
Fakultät für Wirtschaftsinformatik und Wirtschaftsmathematik > Information Systems V: Web-based Systems (Bizer 2012-)
|
Lizenz:
|
Creative Commons Namensnennung 4.0 International (CC BY 4.0)
|
Fachgebiet:
|
004 Informatik
|
Freie Schlagwörter (Englisch):
|
Web Data Integration , Knowledge Base Augmentation , Long-Tail Entities , Weak Supervision , Bootstrapping , Cross-Domain Knowledge Bases , Web Tables
|
Abstract:
|
Data from relational web tables can be used to augment cross-domain knowledge bases like DBpedia, Wikidata, or the Google Knowledge Graph with descriptions of entities that are not yet part of the knowledge base. Such long-tail entities can include for instance small villages, niche songs, or athletes that play in lower-level leagues. In previous work, we have presented an approach to successfully assemble descriptions of long-tail entities from relational HTML tables using supervised matching methods and manually labeled training data in the form of positive and negative entity matches. Manually labeling training data is a laborious task given knowledge bases covering many different classes. In this work, we investigate reducing the labeling effort for the task of long-tail entity extraction by using weak supervision. We present a bootstrapping approach that requires domain experts to provide a small set of simple, class-specific matching rules, instead of requiring them to label a large set of entity matches, thereby reducing the human supervision effort considerably. We evaluate this weak supervision approach and find that it performs only slightly worse compared to methods that rely on large sets of manually labeled entity matches.
|
 | Dieser Eintrag ist Teil der Universitätsbibliographie. |
 | Das Dokument wird vom Publikationsserver der Universitätsbibliothek Mannheim bereitgestellt. |
Suche Autoren in
Sie haben einen Fehler gefunden? Teilen Sie uns Ihren Korrekturwunsch bitte hier mit: E-Mail
Actions (login required)
 |
Eintrag anzeigen |
|
|